Neuroimaging Is The Future of CNS Drug Development
Inside Alto Neuroscience's Efforts to Revolutionize Clinical Trials
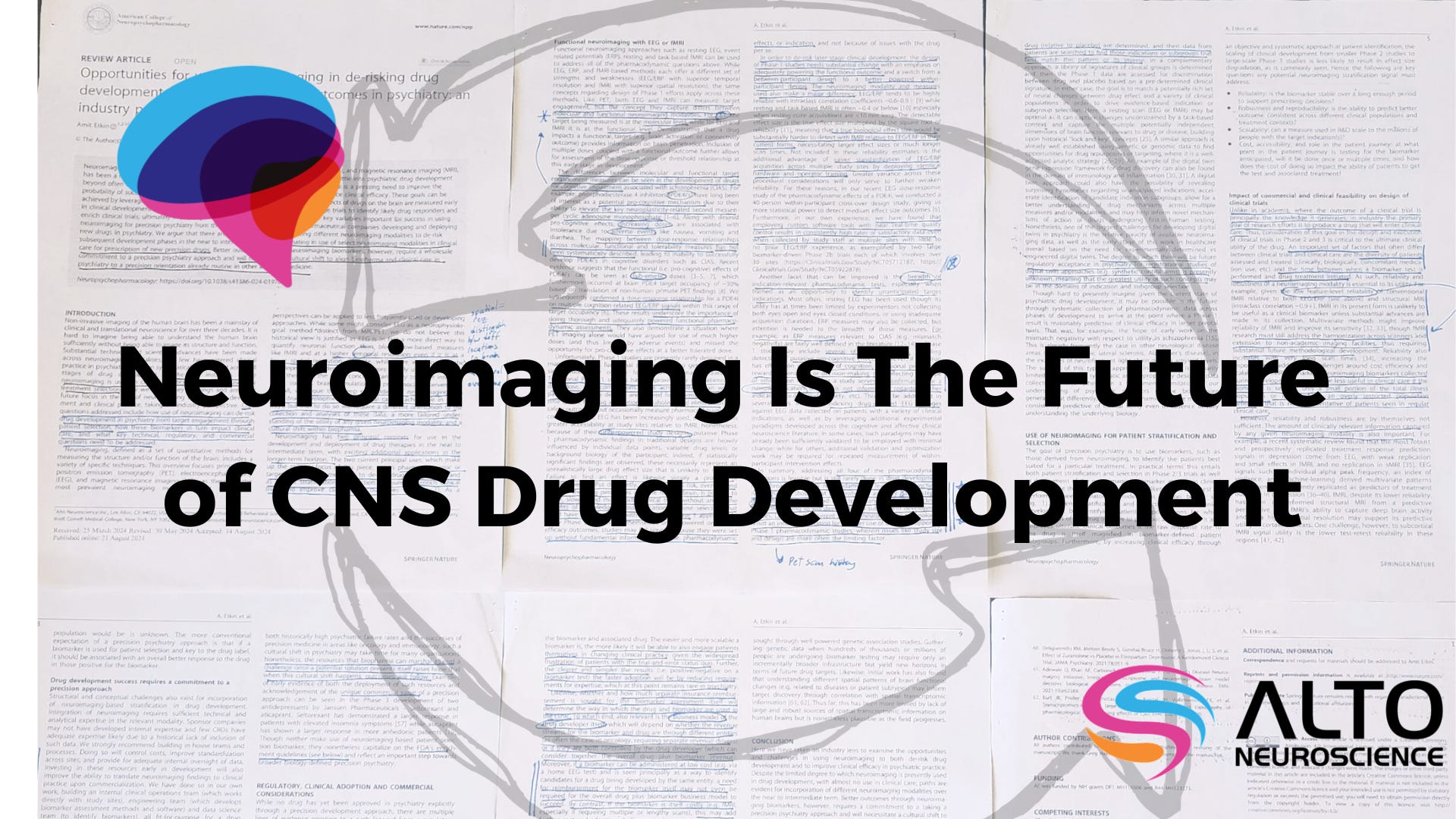
Clinical Trials are top of mind lately. They’re so important, but so antiquated.
Neurotechnology’s opportunity to make clinical research and treatment more effective and efficient for all parties remains largely unrealized across the pharmaceutical industry. Though many life sciences companies have placed early bets on digitization and decentralization, few have built their entire business model on leveraging novel brain health biomarkers in “owned” drug development.
Enter Alto Neuroscience, a psychiatric pharma startup powered by neurotech R&D.
Let’s start with a quick overview of what that entails, from their 2023 10-K:
“Our Platform and differentiated approach aim to disrupt the trial-and-error method that is currently standard practice in CNS drug development and clinical care….Our Platform and approach employ modern tools for measuring human neurobiology together with rigorous data science analytics to discover, and prospectively replicate, biomarker responders, or patients that may demonstrate better clinical response to our novel product candidates. We believe this approach (can) increase the probability of clinical success by magnifying the impact of each product candidate, thereby yielding a differentiated drug profile.”
Alto’s CEO Dr. Amit Etkin, MD PhD, Chief Development Officer Jessica Powell & Chief Medical Officer Dr. Adam J. Savitz, MD PhD recently published a paper titled, “Opportunities for use of neuroimaging in de-risking drug development and improving clinical outcomes in psychiatry: an industry perspective.”
The term “precision neuroscience” is en vogue, making this paper a must-read for any executives interested in nuts and bolts of neurotech’s opportunity to transform two multi-billion dollar industries, starting with clinical trials and bridging patient care.
In this piece, I’ll summarize key takeaways from this paper, along with commercial considerations for biomarker platform development using neurotech, based on a broader sweep of Alto’s publicly shared documents. Here’s what we’ll cover:
Company History
Major Takeaways
Biomarker Development List
Value Flywheel
“Full—Stack” Precision CNS Drug Development *
Inside Alto’s Strategy & Ops: Tech/Data Stack, Companion Dx, R&D Costs
Drug Sourcing Strategy *
* - The starred items are behind the paywall. Paying members will find additional analysis of Alto’s strategy and operations based on their 10-Q filing from August 2024 and their 10-K from last year. If you value original neurotech analysis, you can upgrade or, take advantage of Substack’s reader referral program.
My shameless goal for this piece is to begin a playbook to inspire and facilitate the creation of Alto Clones who will (eventually, collectively) use neurotech as a battering ram to bash paper-survey-based endpoints into yesterday. But even before digital endpoints become the norm, neuroimaging can turbocharge how clinical trials are conducted at nearly every step, unlocking new treatments for patients and families, while generating millions of bucks for bold, smart founders and VCs in the process.
Sound good? Great. Let’s get into it.
Quick Company History
Alto was founded in 2019, based on a decade of Dr. Etkin’s research on neuroimaging’s abilities to predict treatment response at Stanford. After an $8m seed round, they emerged from stealth in 2021 with a $32m Series A led by Christian Angermayer’s Apeiron Investment Group and support from Windham Partners, What If Ventures, Able Partners, and others. At the time they touted 11 “clinical stage assets.”
In early 2023, along with a $25m Series B led by Alpha Wave Ventures, they entered a $35m credit facility with K2 HealthVentures. Just 10 months later, Alto closed a $45m Series C led featuring InVivium Capital, Franklin Templeton, Point72, Alexandria Venture Investments, and Eli Lilly.
By this time they had refined their pipeline down to their current five CNS candidates: ALTO-100, ALTO-202, ALTO-300, ALTO-101, and ALTO-203, which comprise their pipeline across numerous indications today:
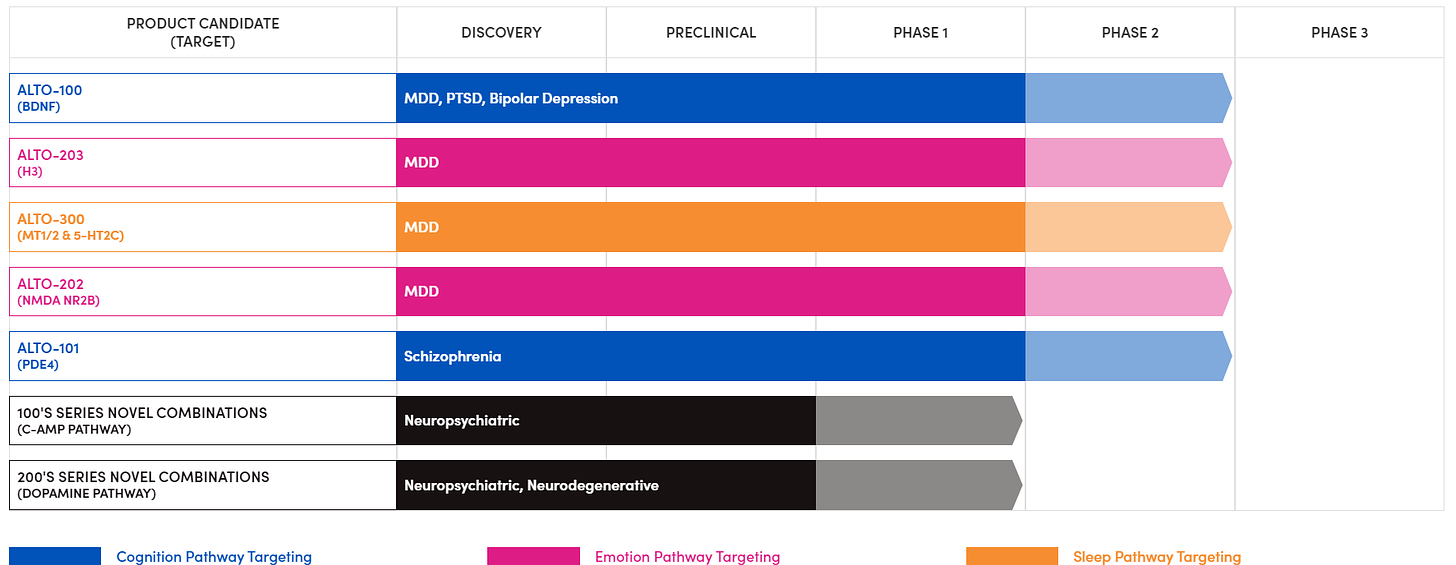
With $145m in the bank, Alto then completed a $129m IPO in February of this year, selling 8 million shares at $16 a pop. The stock price went up to $21.80 and has since leveled off around $11/share at the time of this writing. Finally, Alto brought in another $11.7m in a research funding award from the Wellcome Trust, post-IPO.
There are plenty more details, but the point is that this is the kind of fundraising success most founders can only dream of. So, what’s the secret? Back to that paper.
Major Takeaways
The paper presents “key learnings and areas for future focus in the use of neuroimaging in both drug development and clinical practice, taking an industry perspective.” But what makes this so special is that the vision outlined by Alto’s brain trust applies more broadly to other markets and modalities not covered therein.
For example, outpatient neurology and primary care clinics could benefit from many of the same advances as psychiatric practices. And, to the extent that medical device development suffers from the same paper measures and subjective endpoints as drug trials, neuroimaging’s potential for robust data collection, measure development, patient stratification, and commercial strategy covered below still applies.
By considering three modalities in practice (EEG/ERP, PET, fMRI) the authors illustrate commercial considerations in developing biomarker platforms.
Topline Takeaways:
Despite progress in neuroimaging tools, they remain underused where they are needed most: Quantifying patients responses to drugs during trials, and determining which patients are likely to respond to which treatment.
Underpowered Studies in CNS drug trials, driven by “All-Comer” population selection and “product instead of platform” thinking fail to demonstrate clinical outcomes, sabotaging FDA approval. This is avoidable with neuroimaging-enabled precision for more intentional participant selection, dose selection, drug effects, indication-related measurement, and other considerations.
The Ultimate Vision is a symbiosis between clinical practice and clinical trials, wherein the right patients are more accurately treated in a more timely manner, and recruitment is not a stab in the dark, but an orchestrated, data-driven process. The “flywheel" model explored below will be familiar to anyone with a public health background for whom “learning healthcare systems” ring a bell.
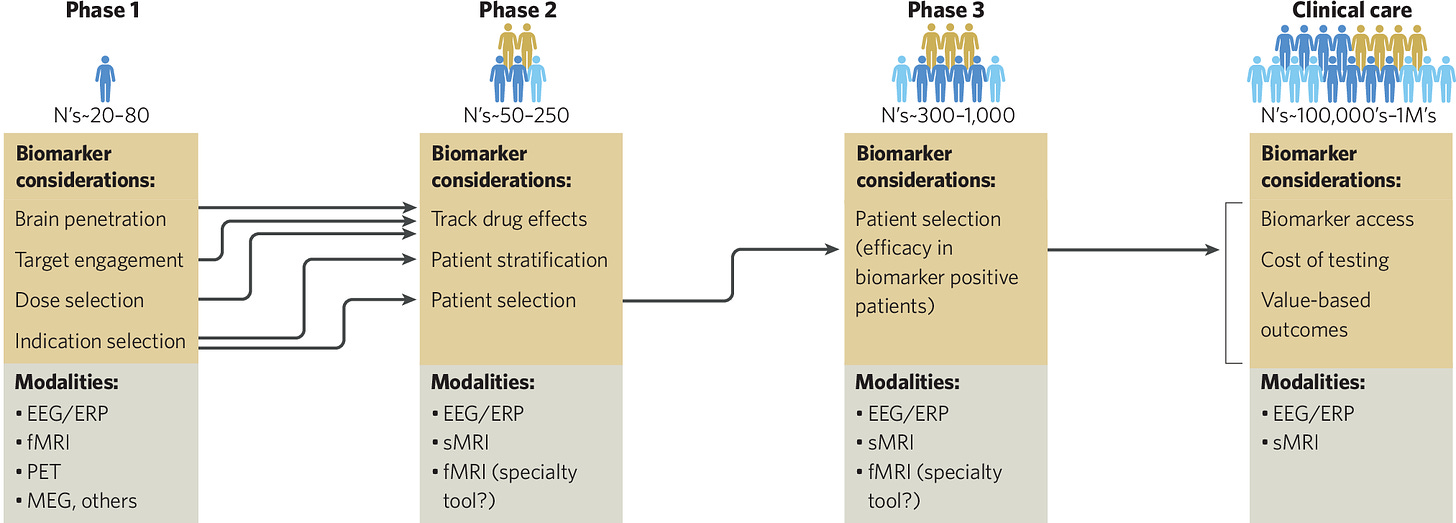
Revenue Strategy: Alto’s conviction is that developing treatments is more important than developing neuroimaging modalities alone, in part because drug development creates an “owned opportunity” for in-market testing with neuroimaging. But this ultimately depends on both the specific modality chosen, and the specific opportunity (market, indication, application, etc.) being pursued.
I won’t mince the paper’s overarching arguments for neuroimaging’s transformative potential, which the authors make quite clearly:
“There is no fundamental reason why functional neuroimaging should not be positioned as a key gating step in decisions on whether and how to advance a drug into clinical trials, since doing so will directly de-risk subsequent development phases….The current state of neuroimaging technologies is not an inherent limitation for broader use of decision-supportive Phase 1 pharmacodynamic studies, wherein issues like study size and design are more often the limiting factor.”
Biomarker Development Checklist
Considerations for biomarker development are broken into two groups: Those related to assessing the drug’s impact, and those related to the pragmatic considerations involved in clinical data collection, from lab to living room.
Pharmacodynamic Measures
Pharmacodynamics involve what a drug does to the body; Think of it as ‘impact calculus’ or dose response.
In the context of neuroimaging for clinical trials, the authors outline four key questions to optimize cost, time, and risks to ultimate regulatory approval.
Brain penetration: does the drug enter the human brain?
Functional target engagement: what impact does the drug have on clinically relevant brain systems and functions?
Dose selection: what is the dose-response relationship on those brain responses, and how does that inform dose selection for clinical trials?
Indication selection: How do the brain effects drive the selection of an indication, or even a biomarker- or clinically defined subgroup within an indication?
Patient Stratification
This refers to sorting patients into tiers based on likelihood of responding to a drug, developing a certain disease, or other risk rating.
Neuroimaging-enabled sorting can help de-risk later stage research in clinical trials that study larger groups of patients, as well as help doctors match the right patient with the right treatment as part of routine clinical care.
With this in mind, the authors offer four additional questions for a biomarker platform strategy:
Reliability: is the biomarker stable over a long enough period to support prescribing decisions?
Robustness and reproducibility: is the ability to predict better outcome consistent across different clinical populations and treatment contexts?
Scalability: can a measure used in R&D scale to the millions of people with the target indication(s)?
Cost, accessibility, and role in the patient journey: at what point in the patient journey is testing for the biomarker anticipated, will it be done once or multiple times, and how does the cost of doing so impact the ability of patients to get the test and associated treatment?
The goal of precision psychiatry is to use biomarkers, such as those derived from neuroimaging, to identify the patients best suited for a particular treatment.
Modality Matters
Neuroimaging technology must be selected not just based on what it can do for a given study, but also the overall operation. The paper’s discussion of key commercial considerations, summarized below, doubles as a strategy roadmap for founders, investors, commercial operators of all stripes.
Cost and Scalability: Since cost and scalability of data collection can “determine utility in the context of similar reliability, robustness, and information content,” these practical factors count as much as purely scientific considerations. This means building data capture protocols that work over the entire course of a treatment journey, and understanding which measures are optimal to collect at the beginning, versus middle or end of a trial.
Reimburse-ability: Perhaps a no-brainer, but how does the company deploying neuroimaging assessments make money? The paper delineates two broad business models: Separating biomarker & drug revenue, versus combining them.
Separate: This model is more commonly seen in oncology and other disease areas where “precision” has taken hold. For Neurotech, look at Beacon Biosignals, Cumulus Neuroscience, and others, who sell their capabilities to Pharma Sponsors.
For neurotech companies trying to generate revenue, strategically this is a trickier decision than it appears, as “whether and how much separate insurance reimbursement is sought for the biomarker assessment itself will determine the way in which the drug and biomarker are used in the clinic.” The PET Scan offers an example of both the promise and limitations of custom R&D (see history sidebar, below)
Combined: This “full-stack” approach is Alto’s model, which we’ll explore in further depth below. By selecting a lower-cost and more accessible screening modality (EEG), Alto reduces overall friction to their broader operation, while powering the ongoing flywheel of biomarker refinement and development.
Other Regulatory and Reimbursement Considerations
“If the biomarker is itself costly this may add further cost and adoption challenges to its use prior to a patient being identified as a likely drug responder and contributing to this likely larger source of revenue.”
“For such costly biomarkers, an additional challenge is the adoption of Current Procedural Terminology (CPT) codes and Medicare reimbursement, such as happened for beta amyloid PET imaging.”
“An additional factor to consider is that a neuroimaging patient selection marker will likely also require a parallel clearance as software as a medical device (for scoring the biomarker), along with a potential clearance of the neuroimaging device itself if not already cleared. Thus, a neuroimaging-based enrichment-driven drug development effort must include engaging with both the drug and device divisions within the FDA. These factors may increase cost and complexity required for precision drug development.”
The easier a biomarker is to use, the more scalable it is with clinicians, research sites, and eventually consumers. Simple results (e.g. “positive/negative”) and reliability for data capture across different settings are some key considerations.
The paper employs three neuroimaging modalities to illustrate this in practice: PET, fMRI, and EEG.
Well, sort of. A quick aside:
What appears at first glance like a reasonable pros/cons comparison reveals itself on successive readings as a thinly veiled argument that “EEG is best.” Whether or not you agree, this is perfectly defensible given Alto’s business strategy and Dr. Etkin’s body of work, even though it may ruffle feathers in academic circles. Suffice to say, Alto has a horse in this race, so for those seeking objective head-to-head analysis of EEG vs other modalities, this ain’t the paper for you.
Ok, so why EEG? As per Alto: “EEG systems have themselves rapidly advanced over recent years with technology such as dry electrodes, ambulatory at-home uses, as well as existence of a number of low-cost consumer-grade devices. Thus, it is reasonable to expect that home-based EEG assessments can be done routinely at scale with device manufacturing costs <$1,000 in the near term. This cost point, coupled with neuroimaging that can be self-administered by the patient through guidance by tailored acquisition software, additionally enables the potential for repeated or longitudinal assessments (see section below) which itself opens new horizons for biomarker utility in psychiatry.”
Beyond stating the obvious that EEG is cheaper, faster, easier to use compared to PET Scans and fMRI, I’ll point you to the linked notes below for 1) a fun list of times the authors diss fMRI; and 2) The PET Scan’s interesting commercial history.
To state the obvious: Selection of modality matters quite a bit, i.e. EEG can be useful for functional measures of cognitive states, while PET is better suited to molecular level measurements, and authors note the cost and development time of a new PET tracer means it is not commonly used in de-risking drug trials.
One last note here is that at the earliest stages of research, the common hammer-seeks-nail approach to capturing certain desired signals can backfire by missing the opportunity to understand the drug more fully: “If the drug has effects on other facets of cognition, emotional processing, reward responsivity, or decision-making, not collecting this type of information means that a study serves as confirmatory for a narrow indication set but is not informative regarding other indications (e.g. mood, anxiety, etc).”
Regardless of modality, the paper’s merit is that its broader decision calculus applies to many neurotechnologies, including tools (and combos!) not mentioned here.
(As a bonus for anyone interested in further analysis of biomarker translation, with a focus on EEG vs. sMRI and fMRI, see the chart on page 4 of this OTHER recent paper by Dr. Etkin.)
The Value Flywheel
In-market adoption of neuroimaging biomarkers remains slow for two reasons: First, a shortage of “well-validated measures” to drive millions of dollars of decision-making at conservative pharma companies, and second, poor alignment between whether/how well a given neuroimaging modality can detect a drug in the human body, and whether/how well that drug actually works in treating the target indication.
My understanding is that the market remains stuck in a version of the streetlight effect: Being able to see something in a test doesn’t mean you’ve found what you seek. Alto’s bet is that funding and standardizing repeated neuroimaging feedback loops at successive stages of drug development (Fig 2 below) will produce medicines that are more likely to gain FDA approval.
The ultimate goal of this approach is to design a way for CNS drugs to work better in patients to whom they are prescribed:
“The more conventional expectation of a precision psychiatry approach is that if a biomarker is used for patient selection and key to the drug label, it should be associated with an overall better response to the drug in those positive for the biomarker.”
If this is all too complicated, just think about it in terms of feedback loops.
A helpful analogy for me was to think of this as “3-D printing” biomarkers over time, or in this case, several development cycles. This starts with tracking pharmacodynamic effects of a drug in a Phase 1 trial, using those data to inform the design of Phase 2 protocols, then closing the loop by informing the model of patient responses, gathered via subsequent neuroimaging.
Early feedback loops: Especially at the investigational stage, this method can help isolate signals that inform strategy on optimal dose sizing (word of the day: sub-emetic) and measure selection: “Standardizing this approach over multiple clinical development programs will indicate which pharmacodynamic effects are most predictive for which sorts of clinical outcomes and which may be very helpful for addressing some early-stage questions but are not directly predictive of clinical efficacy.”
But the authors are clear that the real value proposition of this approach is in informing patient selection for later stage trials, learning from their clinical outcomes, and developing the ability to prescribe drugs more effectively in practice.
Longer feedback loops, or those that happen farther down from initial pharmacodynamic measurement can mean better prediction of patient response, which can mean better cohort selection for later stage trials, which can mean boosting likelihood of regulatory approvals.
Two key concepts worth understanding here are Enrichment and Prospective Replication.
Enrichment: As defined by the FDA in their recent guidance, “Enrichment is the prospective use of any patient characteristic to select a study population in which detection of a drug effect (if one is in fact present) is more likely than it would be in an unselected population.”
“While suggested designs differ, they generally focus analyses on patients positive for the biomarker (enrichment marker), which need to be well-enough defined and powered as the primary efficacy population.”
Authors point to two non-neuroimaging enabled refinement models in antidepressant R&D by Janssen, seltorexant and aticaprant which research suggests may work more effectively in specific sub-groups of patients with elevated insomnia and anhedonia, respectively. Identifying responders with neurotech is a big and essentially untapped opportunity in this vein.
Prospective Replication: Once a biomarker has been created based on neuroimaging data of a) a drug’s effects and b) a clinical response, it can be used to screen a fresh batch of patients, or “a completely independent patient cohort not used in any part of the analyses to determine the biomarker.” (See Fig 3).
The authors acknowledge this is more expensive and challenging from a data acquisition standpoint compared to “conventional cross-validation approaches used in neuroimaging ML models,” but also assert it is “likewise well-documented that the kind of machine learning analyses increasingly used to develop candidate biomarker signals can overfit on the data on which they are trained and not generalize to other samples.”
Other Market-Relevant Tidbits:
“In drug development, neuroimaging data are rarely collected in Phase 2 studies and even less frequently in Phase 3 studies, leading to even less available data. However, given the expectation in industry that analysis of clinical trial data be directed through pre-specified statistical analyses plans, use of independent data to prospectively test the ability of a biomarker to predict outcome can be conceptualized under a similar framework. “
“In our own work, we have required that candidate patient selection biomarkers be prospectively replicated in an independent cohort of patients receiving the study drug. This was already successful in two programs in depression. Both of these have advanced to Phase 2b trials wherein patients are selected based on a combination of their diagnosis (major depressive disorder) and status on a biomarker test comprised of either performance in a test of memory or a specific EEG pattern. (See ALTO-100 and ALTO-300)
“Full—Stack” Precision CNS Drug Development
With parameters in place to select and configure the right neurotech for R&D, let’s zoom out and revisit the business Alto is building. After that, we’ll pin down some numbers on what it would take to replicate - or remix - their commercial strategy.